AI-Driven Intent Recognition for Lumbar Support Exoskeleton
Professor Introduction
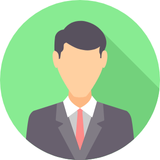
J. Y | Ph.D. in Computer Science
Home Institute:Harbin Institute of Technology
[ Research Interests ] Bionic Robots, Artificial Intelligence, Intelligent Equipment
[ Additional Experience ] Senior Robotics Expert with over ten years of experience in robotics development;Participated in several National Natural Science Foundation and National 863 Program projects
[ Publications ] IEEE Member, Visiting Scholar at the University of Utah;Principal investigator of multiple national and provincial projects with extensive teaching and research experience
Project Description
This project focuses on designing and developing a lumbar support exoskeleton to assist users in reducing the physical strain during bending tasks, thereby protecting the occupational health of manual laborers. The project involves designing the exoskeleton based on human body dimensions, simulating movements, and optimizing the structure using 3D modeling software (SolidWorks). It then uses electromyographic (EMG) signals and AI algorithms to recognize human motion intentions, controlling the servo-driven exoskeleton's movements (programmed in Python). Finally, a prototype will be manufactured, debugged, and tested to determine the optimal control parameters for assistance, with a quantitative analysis of the support effect on lumbar joints (data processing and analysis using Python).
Project Keywords
Project Outline
Part 1 : Introduction to Exoskeletons and Lumbar Support
• Overview of Exoskeleton Technologies and Applications
• Importance of Lumbar Support in Occupational Health
• Introduction to AI Intent Recognition and EMG Signals
Part 2 : Research Objectives and Hypotheses
• Investigating the Enhancement of Tumor Segmentation by Integrating Multimodal MRI Images
• Developing and Validating Deep Learning Models for Tumor Segmentation
Part 3 : Review of Current Research and Methods
• Review of Existing Methods for Brain Tumor Segmentation
• Identification of Gaps and Limitations in Current Technologies
Part 4: Data Collection and Preprocessing
• Collection of Multimodal MRI Brain Tumor Datasets
• Preprocessing Techniques for Image Enhancement and Normalization
• Data Augmentation Strategies to Improve Model Robustness
Part 5 : Development of Deep Learning Models
• Building Convolutional Neural Networks (CNNs) for Tumor Segmentation
• Incorporating Multimodal Data into the CNN Architecture
• Setting Up Training Parameters and Loss Functions
Part 6 : Model Training and Evaluation
• Training the Deep Learning Models on Multimodal MRI Data
• Evaluating Model Performance Using Metrics such as Dice Coefficient and IoU
• Fine-Tuning Hyperparameters to Optimize Model Accuracy
Part 7: Comparative Analysis and Model Optimization
• Comparing the Performance of Different Deep Learning Architectures
• Analyzing the Impact of Multimodal Integration on Segmentation Accuracy
• Iteratively Optimizing the Model for Better Performance
Part 8: Results and Discussion
• Graphical Representation of Segmentation Results and Performance Metrics
• Interpretation of Results and Discussion of Clinical Implications
• Comparison with Existing Segmentation Methods and Discussion of Advantages and Limitations
Part 9: Conclusion and Future Directions
• Summary of Key Findings and Their Significance
• Identification of Research Limitations and Suggestions for Future Research
• Recommendations for Practical Applications in Clinical Medicine
Part 10: Reporting and Presentation
• Writing a Detailed Research Report with Clear Structure, Concise Language, and Accurate Data Presentation
• Preparing and Delivering a Clear and Engaging Oral Presentation of Research Background, Methods, Results, and Conclusions
Suitable for
High School Students:
• Interest in AI and Medical Imaging: Students with a strong interest in artificial intelligence, machine learning, and medical imaging technologies.
• Basic Knowledge : Students with a basic understanding of programming and image processing concepts.