Clustering and Covariance Structure Analysis of Heterogeneous Longitudinal Data
Professor Introduction
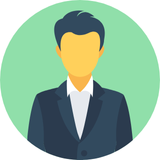
J. Y | Ph.D. in Statistics
Home Institute:Sichuan University
[ Research Interests ] Longitudinal data and survival analysis, covariance estimation, mixed models, variable selection methods
[ Teaching Experience ] Lecturer, Department of Statistics and Data Science, Chongqing University of Technology
[ Additional Experience ] Member of the Multivariate Analysis Branch of the China Field Statistics Research Association, participated in many important research projects at home and abroad
Project Description
This project addresses the clustering of heterogeneous longitudinal data and the impact of different covariance structures on clustering outcomes. It aims to provide a comprehensive understanding of how various covariance structures and clustering methods can affect the accuracy and validity of clustering results. By applying these techniques to heterogeneous longitudinal data, students will learn to analyze and interpret the distinct trajectory patterns of different subgroups within the data.
Project Keywords
Project Outline
Part 1 : Introduction to Longitudinal Data Analysis
• Basics of longitudinal data and its importance
• Common challenges in analyzing longitudinal data
• Overview of heterogeneity in longitudinal data
Part 2 : Covariance Structures in Longitudinal Data
• Different types of covariance structures
• Methods for estimating covariance structures
• Impact of covariance structures on data analysis
Part 3 : Clustering Methods for Longitudinal Data
• Overview of clustering techniques
• Applying clustering methods to longitudinal data
• Evaluating the performance of clustering methods
Part 4 : Impact of Covariance Structures on Clustering
• Analyzing the influence of different covariance structures on clustering results
• Comparative studies of clustering accuracy and validity
• Case studies and practical applications
Part 5 : Advanced Topics and Applications
• Advanced methods in longitudinal data clustering
• Practical applications in various fields such as biostatistics and social sciences
• Future research directions and challenges
Suitable for
• High School Students interested in longitudinal data analysis and clustering methods
• Graduate students and advanced undergraduates in statistics and data science