Performance Study of Data-Driven Reynolds Averaged Modeling under Different Prandtl Numbers
Professor Introduction
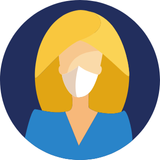
X. X | Ph.D. in engineering
Home Institute: University of Melbourne
[ Research Interests ] Application of machine learning in scientific computing、Turbulence modeling、Structural wind engineering
[ Additional Experience ] Member of the Australian Fluid Mechanics Society、Member of the Chinese Society of Mechanics、Participation in multiple domestic and international research projects
Project Description
This research project aims to analyze the flow fields under different Prandtl numbers using machine learning methods. By constructing Reynolds-averaged models for fluid and heat transfer, the project seeks to enhance the prediction accuracy of Reynolds-averaged models for flow fields at various Prandtl numbers.
The Prandtl number is a dimensionless number that characterizes the relative thickness of the momentum and thermal boundary layers in a fluid flow. Accurate modeling of flow fields under different Prandtl numbers is crucial for various engineering applications, including heat exchangers, aerodynamic heating, and environmental fluid dynamics.
The project will leverage data-driven approaches, specifically machine learning techniques, to address the closure problem in Reynolds-averaged Navier-Stokes (RANS) equations. By integrating machine learning with traditional turbulence modeling, the project aims to develop more accurate and robust models for predicting flow behavior under different thermal conditions.
Project Keywords
Project Outline
Part 1 : Introduction to Prandtl Numbers and RANS Modeling
• Overview of Prandtl numbers and their significance in fluid dynamics.
• Introduction to Reynolds-averaged Navier-Stokes (RANS) equations and the closure problem.
• Importance of accurate RANS modeling for engineering applications.
Part 2 : Machine Learning in Scientific Computing
• Overview of machine learning techniques used in scientific computing.
• Application of machine learning in turbulence modeling.
• Review of existing data-driven approaches for RANS modeling.
Part 3 : Data Collection and Preprocessing
• Identification of relevant datasets for different Prandtl numbers.
• Data preprocessing techniques, including normalization and feature extraction.
• Handling of missing data and data augmentation strategies.
Part 4 : Model Construction and Training
• Development of machine learning models for RANS closure terms.
• Training and validation of models using collected datasets.
• Hyperparameter tuning and model optimization.
Part 5 : Model Evaluation and Analysis
• Evaluation of model performance using standard metrics.
• Comparison of machine learning-based models with traditional RANS models.
• Analysis of model predictions for different Prandtl numbers.
Part 6 : Case Studies and Practical Applications
• Application of the developed models to real-world engineering problems.
• Case studies demonstrating the improved prediction accuracy of the models.
• Discussion of practical implications and potential areas for further research.
Suitable for
• High School Students :In fields such as fluid dynamics, heat transfer, and computational mechanics, who are interested in the application of machine learning in scientific research.